The European Association for Data Science organises a summer school
Generative AI
Tuesday June 18th to Friday June 21th 2024 in Luxembourg.
As we had a very positive echo of the Summer School 2023 “Data Science for Explainable and Trustworthy AI”, another one will be organised in 2024 on the topic of “Generative AI”.
The rise of Generative AI, especially with the advancements in Large Language Models (LLMs), marks a transformative era in artificial intelligence that is expanding across all disciplines. LLMs aim to bridge the communication gap between machines and humans, paving the way for models that can grasp the nuances of human language and generate outputs in various formats that mimic human cognition and creativity.
The critical moment for Generative AI came with the adoption of neural networks, particularly transformer-based architectures, which have become its backbone. These models stand out for their profound ability to digest and learn from extensive corpora and datasets, and also to generate original, contextually rich content. But we are just at the beginning. The emerging models present challenges related to ethics, reliability, the way we experiment with these models, the scope of their inferences, their applications to more specific domains, etc. All this has created a vibrant field of work and opens the doors to a community that we hope will find the right forum in this Summer School.
The Summer School 2024 will take place at the venue of
Maison d’Accueil (Convent of the Franciscan Sisters)
50 avenue Gaston Diderich
L – 1420 Luxembourg-Belair
Public Event
The summer school will be preceded by a public event on Tuesday, June 18th starting 15:00 pm.
At the heart of the public event is the Sabine-Krolak-Schwerdt-Lecture, in memoriam of EuADS’ founding president. This will be held by Peter Flach, (University of Bristol, UK). Participation in the public event is free of charge. Please note that registrations for the public event are still possible.
Agenda
15h00– 16h00 | Registration and Coffee |
16h00– 17h00 | Opening and Welcome Eyke Hüllermeyer EuADS President |
17h00– 18h30 | Sabine Krolak-Schwerdt Public Lecture Data Science in the time of ChatGPT — Why AI isn’t solved, and how data science can help Peter Flach University of Bristol, UK |
18h30 | Welcome Reception |
from the 18th – 21st of June 2024. A limited number of rooms for smaller travel budgets will be available at the premises. Attendees are, however, not obliged to take residence there.
All public transport in Luxembourg is for free. You can look up all schedules in the app: Mobilitéit.lu
Data Science in the time of ChatGPT -- Why AI isn't solved, and how data science can help
Peter Flach (University of Bristol, UK)
Looking at a list of major milestones in artificial intelligence (AI) over the last quarter century, it is hard to escape the impression that the rate of innovation in AI is accelerating dramatically if not exponentially: in 1997 IBM’s Deep Blue beats chess world champion Garry Kasparov in a six-game match, in 2011 IBM’s Watson wins against two human experts at the Jeopardy quiz show, in 2016 DeepMind’s AlphaGo beats top Go player Lee Sedol in a five-game match, in 2020 DeepMind’s AlphaFold accurately predicts 3D protein structures, and today generative AI models such as OpenAI’s ChatGPT can generate convincing, human-like text allowing them to pass academic exams and produce executable computer code from text prompts.
However, objectively speaking the above argument is fraught with difficulties, for a wide range of fundamental reasons. One is that, in competitive settings such as the first three, what really matters is not just the observed outcome but a robust estimate of its likelihood: if we re-ran these contests a number of times, what distribution of wins and losses would we expect? Another is that in many cases the intended outcomes are ill-defined: what does it mean to accurately predict protein structures? How do we measure human-likeness of text? Does the AI system convey a degree of confidence with its outputs? Can it explain its reasoning, and take corrections or feedback into account?
As I will show in this talk, this is where data science can help. I will review classical and recent work in producing calibrated probability estimates which directly addresses issues around confidence and distribution of outcomes. In the spirit of Sabine Krolak-Schwerdt, who held a chair in Educational Measurement, I will then explore the links between performance assessment of machines and human evaluation. Taking inspiration from cognitive science and psychometrics will allow us to come up with more meaningful measuring instruments, standards and benchmarks and move away from the overly simplistic league table approach that has been dominant in machine learning and AI for too long. In the foundational subject of AI performance evaluation, it takes the truly interdisciplinary outlook of data scientists to make meaningful and tangible progress.
Speaker's WebpageTopics and Presenters
The schedule for the summer school including speakers and topics:
Tuesday, June 18th | tba | Sabine-Krolack-Schwerdt-lecture: Data Science in the time of ChatGPT — Why AI isn’t solved, and how data science can help | Peter Flach (University of Bristol, UK) |
Wednesday, June 19th | 9:30 a.m. to 1 p.m. | Machines That Speak and Imagine: The Role of AI in Audio and Image Generation | Ian Sosa (NeuralWave Technology) |
2:30 p.m. to 6 p.m. | LLMs: Foundations, Advancements, and Ethical Considerations | Thomas Arnold (University of Darmstadt, DE) | |
Thursday, June 20th | 9:30 a.m. to 1 p.m. | Generative AI for Data Analytics | Artur Guja (Data 3.0 Ltd, UK) |
2:30 p.m. to 6 p.m. | Robust Evaluation of Generative AI | Marko Tešić (University of Cambridge, UK) | |
Friday, June 21th | 10:00 a.m. to 12:30 p.m. | Generative AI for Health | Ewa Szczurek (University of Warsaw, PL & Helmholtz Munich, DE ) |
For details see below!
Wednesday, June 19th
Machines That Speak and Imagine: The Role of AI in Audio and Image Generation
Ian Sosa (NeuralWave Technology)
As we venture into the era of artificial intelligence, Generative AI models—particularly those specializing in audio and image generation—are emerging as societal and industry game-changers. These models are not just reshaping the way we perceive and interact with digital content, but they are also redefining the boundaries of what we call creativity and innovation.
In this course, we will delve into the world of these complex models. We will demystify their architectures, explore some of the sophisticated training techniques that bring them to life, and study the fascinating process through which machines learn to synthesize speech and generate images.
Generative AI models are opening up a world of possibilities across various industries. In entertainment, they are revolutionizing content creation by generating unique music tracks and designing artwork that pushes the boundaries of creativity. In healthcare, they are enhancing medical training and surgical planning through the generation of realistic simulations and 3D models. In the field of communication, they are synthesizing human-like speech, transforming our interactions with AI and making them more natural and intuitive.
However, this paradigm shift is not without its challenges. The potential of Generative AI models to generate misleading or inappropriate content raises significant ethical and societal concerns. We will confront these issues head-on, understand their roots, and discuss proactive strategies to mitigate them. This is crucial to ensure that we harness the power of these models responsibly and ethically.
Beyond theoretical discussions, this course will also feature practical, hands-on exercises. These will provide a unique opportunity to witness first-hand how these models operate, offering a deeper understanding of the intricacies of this technology. Through this approach, we aim to provide a balanced perspective on Generative AI models. By exploring their transformative potential and addressing the risks and responsibilities associated with their use, we can better equip ourselves to navigate the future of AI-driven digital content.
Speaker's WebpageLLMs: Foundations, Advancements, and Ethical Considerations
Thomas Arnold (University of Darmstadt, DE)
Large Language Models (LLMs) have emerged as a transformative force in the world of artificial intelligence, captivating researchers and the public alike with their impressive capabilities. This tutorial aims to demystify these complex systems, providing a thorough understanding of their foundations, advancements, and the ethical considerations that accompany their power.
We begin by establishing a solid foundation in the core principles of LLMs. We delve into their architectural design, exploring the intricate neural networks that power their learning capabilities. We then unpack the training methodologies that enable LLMs to process and learn from massive amounts of data, shaping their ability to perform a diverse range of tasks.
Having established the fundamental building blocks, we transition to the exciting realm of LLM applications. We showcase their remarkable potential in various domains, including: Natural Language Generation: Witness how LLMs can craft human-quality text, from generating creative fiction to producing informative summaries of factual topics.
Machine Translation: Explore how LLMs bridge the communication gap between languages, enabling seamless translation across diverse tongues.
Question Answering: Discover how LLMs can act as intelligent assistants, providing insightful answers to complex queries posed in natural language.
However, the power of LLMs is not without its challenges. As we delve deeper, we critically examine the ethical landscape surrounding these models. We explore potential biases that can lurk within their training data, leading to unfair or discriminatory outputs. We discuss the importance of responsible development and deployment to mitigate these risks, ensuring that LLMs are used ethically and for the benefit of society.
Thursday, June 20th
Generative AI for Data Analytics
Artur Guja (Data 3.0 Ltd, UK)
In the dynamic world of data science, Generative Artificial Intelligence stands out as a transformative force for data analysis. This tutorial aims to familiarize you with Generative AI tools and demonstrate their potential to streamline your data analytics endeavors. The format blends a semi-interactive lecture with practical exercises.
We will begin with a crucial aspect of any data project: ensuring data quality. Addressing typical challenges in data cleaning and preprocessing, we will illustrate how Generative AI can automate these processes, ensuring your projects are fueled by high-quality data. We will also weigh the advantages and consider the risks associated with using Generative AI for generating Synthetic Data.
Next, we will delve into how Generative AI eases the complexities of statistical analysis. You will learn how these AI tools can aid in selecting appropriate statistical methods, writing code, and assist in making sense of what the numbers are telling us. Finally, we will further explore the application of Generative AI in interpreting results and offering actionable recommendations.
However, responsible usage of Generative AI is paramount. Therefore, we will explore best practices for applying Generative AI in data analysis, aiming to avoid common pitfalls and recognize the model's limitations, including occasional hallucinations and acquiescence bias. We will also critically examine the risks associated with Generative AI, such as issues with safety, transparency, interpretability, reproducibility, and ethical concerns, which will be a vital component of our discussion.
The tutorial is designed for data science enthusiasts eager to delve into the realm of Generative AI. Hands-on activities will predominantly involve ChatGPT and a Python-enabled Jupyter Notebook. While prior Python knowledge is not mandatory, familiarity with coding and a problem-solving mindset will be beneficial. Expect an interactive session, so drink your coffee beforehand, roll up your sleeves and come prepared to participate actively.
Robust Evaluation of Generative AI
Marko Tešić (University of Cambridge, UK)
In recent years, the landscape of artificial intelligence has been markedly transformed by the advent of Generative AI, showcasing an unprecedented explosion in both the performance at specific tasks and the apparent expansion of general-purpose capabilities. This remarkable progress has not only redefined the boundaries of what AI systems can achieve but also introduced a myriad of unique challenges in evaluating such systems. Traditional machine learning evaluation metrics, often task-oriented and performance-based, fall short when faced with the nuanced and broad-ranging capabilities of generative AI. This necessitates a shift towards estimating the capabilities of AI systems, especially those designed for general-purpose applications, to ensure their suitability for situations and roles that demand specific cognitive skill levels or capabilities. The inherent complexity of these AI systems calls for an evaluation paradigm that can grasp their multifaceted nature and anticipate their fit in real-world scenarios requiring nuanced and robust capabilities. Methodologies and techniques inspired by the cognitive sciences present a more fitting approach than conventional task-oriented benchmarks.
One promising solution to this challenge is the Measurement Layouts framework. This approach utilises large hierarchical Bayesian Networks to infer the capabilities of AI systems in a structured and comprehensive manner. By leveraging domain knowledge about the tasks in question and the demands specific instances require for success, the framework allows for a detailed analysis of an AI system's capabilities and limitations, as well as providing predictive power over future performance on unseen task instances.
In this tutorial, we aim to shed light on the limitations of traditional machine learning techniques in evaluating generative AI and underscore the importance of integrating insights from the cognitive sciences. We will provide an introduction to the Measurement Layouts framework, demonstrating its efficacy in facilitating nuanced evaluations of AI systems. Participants will gain hands-on experience with the framework, learning how to apply it to assess different types of AI systems and make powerful inferences about their potential applications and limitations.
Speaker's WebpageFriday, June 21st
Generative AI for Health
Ewa Szczurek (University of Warsaw, PL & Helmholtz Munich, DE )
In this course, we will explore the transformative impact of Generative AI on the life sciences, with a focus on its revolutionary applications in drug discovery. Generative models are poised to streamline the initial, labor-intensive phase of molecule design. By training on existing compound data, these models can generate novel molecules with desired properties, significantly enhancing efficiency. For optimal performance, it is crucial that these models accurately capture both the data distribution and their intrinsic properties.
I will introduce a specific class of generative models: Variational Autoencoders (VAEs) and their conditional variants, examining them from a probabilistic standpoint. I will cover the principles of variational inference, the probabilistic graphical introduction of VAEs and their loss function. Following this theoretical framework, I will present Generative AI models developed in my lab and by other researchers. These models focus on the conditional generation and prediction of antimicrobial peptides, as well as the generative and predictive modeling of small molecules.
Speaker's WebpageFees and Registration
For EuADS members the fee for participating in the summer school is 150€.
For non-members the fee is 200 € with a free EuADS-membership for 2024.
To ensure an interactive experience the number of participants is limited, so early registration is strongly recommended. Please register by
1. Sending an email with your personal details to contact@euads.org, with reference to EuADS Summer School 2024 Generative AI.
2. Transferring the amount (participation fee only or participation fee and room reservation) to
Account holder: EuADS (European Association for Data Science)
Bank: Banque et Caisse d’Epargne de l’Etat, Luxembourg
IBAN: LU47 0019 4655 6967 1000
BIC: BCEELULL
Please mention in the payment details field:
“EuADS Summer School 2024_Participation Fee” or “EuADS Summer School 2024_Participation Fee and Room Reservation”
The participation fee must be transferred before the 1st of June 2024.
You will receive a confirmation mail as soon as the money has arrived on the EuADS
account. Without payment until the 1st of June 2024, your reservation is cancelled, and the room will be allocated to the next person on the waiting list.
Organisers:
- Serge Allegrezza (STATEC, Luxembourg; EuADS Treasurer)
- Peter Flach (U of Bristol, UK; EuADS Vice-President)
- Tim Friede (U of Göttingen, Germany)
- Nils Hachmeister (Germany; EuADS Vice-President)
- Eyke Hüllermeier (LMU Munich, Germany; EuADS President)
- Berthold Lausen (U of Essex, UK)
- Victoria Lopéz Lopéz (CUNEF Universidad, Spain)
- José Raúl Romero (U of Cordoba, Spain)
- Denise Schroeder (STATEC, Luxembourg)
- Katharina Weiß (Bielefeld University, Germany)
Rooms and Catering
There will be rooms available at the premises:
7 double rooms
2 single beds for 2 persons each room 330 EUR per room for 2 persons for 3 nights.
In order to avoid misunderstandings, please clearly mention the name of the person with whom you want to share the double room.
The rooms are available from the 18 – 21th of June 2024 at the following email: denise.schroeder@ext.statec.etat.lu
All bookings must be made before the 20th of May 2024. The payments for the rooms must be transferred before the 1st of June 2024.
The catering (breakfast, lunch, dinner and cocktail) will be offered by our sponsors
Further Accomodations
From the following hotels you can walk to the location:
Hôtel Parc Belle-Vue – Goeres Hotels Luxembourg
Hôtel Parc Belair – Goeres Hotels Luxembourg – Groupe Hôtelier
Of, for smaller budgets:
Sponsors

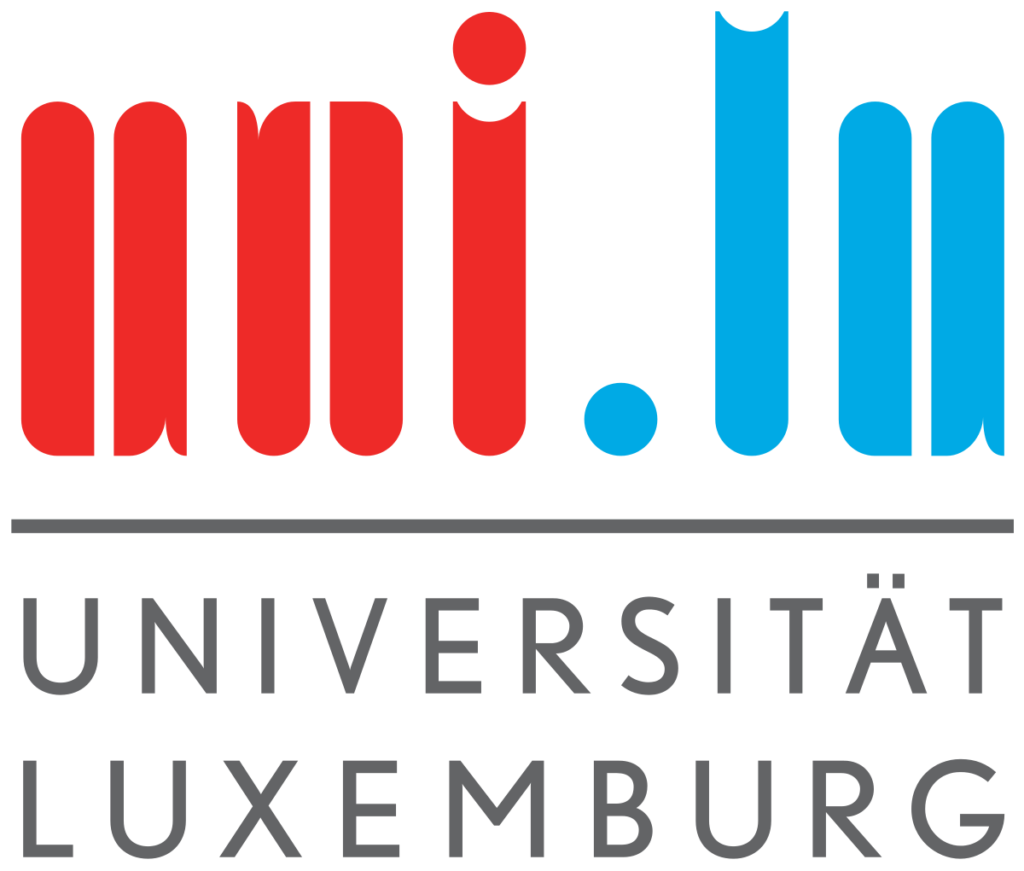




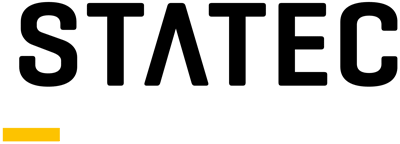